Human-like facial experession in DCNN
Atifical Neural Network as a tool to probe the necessity of experience in facial expression representation
Face identity and expression have been traditionally viewed as separate process in the brain, evidenced by patient cases and neuroimaging studies (FFA/OFA VS STS). However, incresing evidence suggests that both informantion can be decoded from the ROIs (for example, FFA/OFA have been regarded as face identity region, but can also process facial expression information).
In this paper, we examine whether the interdependence of face identity and expression is nessary from a computatioanl point of view. We used VGG-face, a network optimized for facial identity recognition, as the model to simulate cognitive process in the brain(Fig. 1).
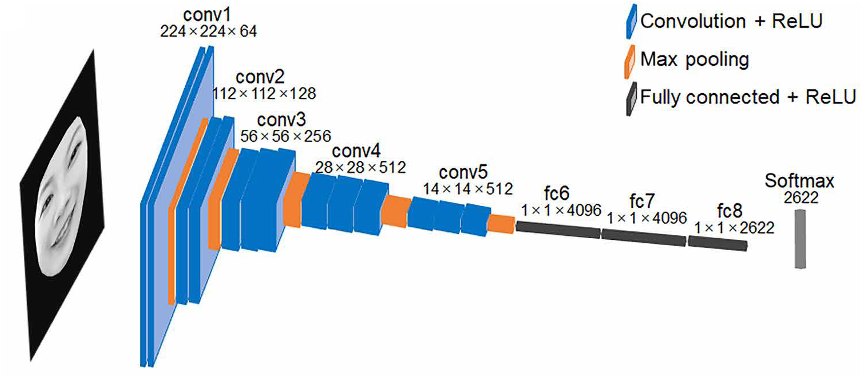
We found expression-selective units in VGG-face, and more importantly, those units were human-like, which was evidenced by similar confusion matrix(Fig. 2) compared to human subjects and clear categorical perception of morphed expression continua, a hallmark of human expression recognition property.
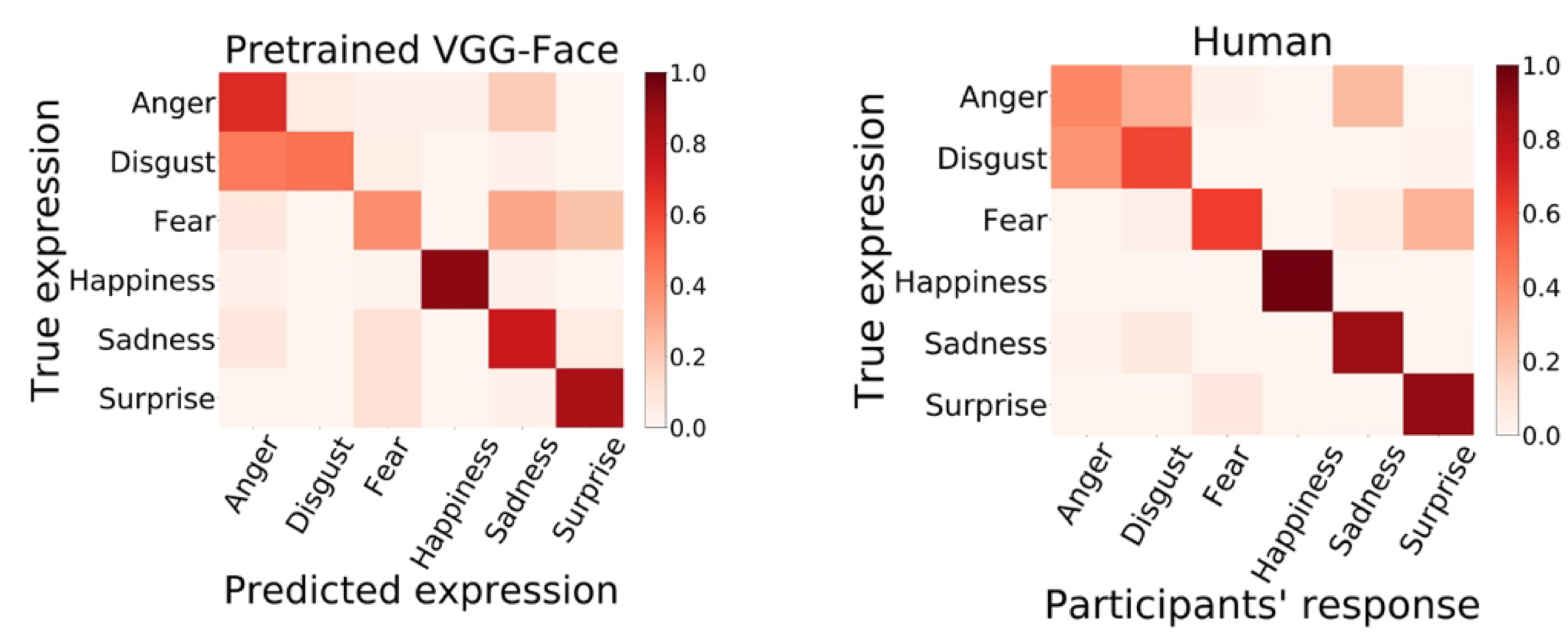
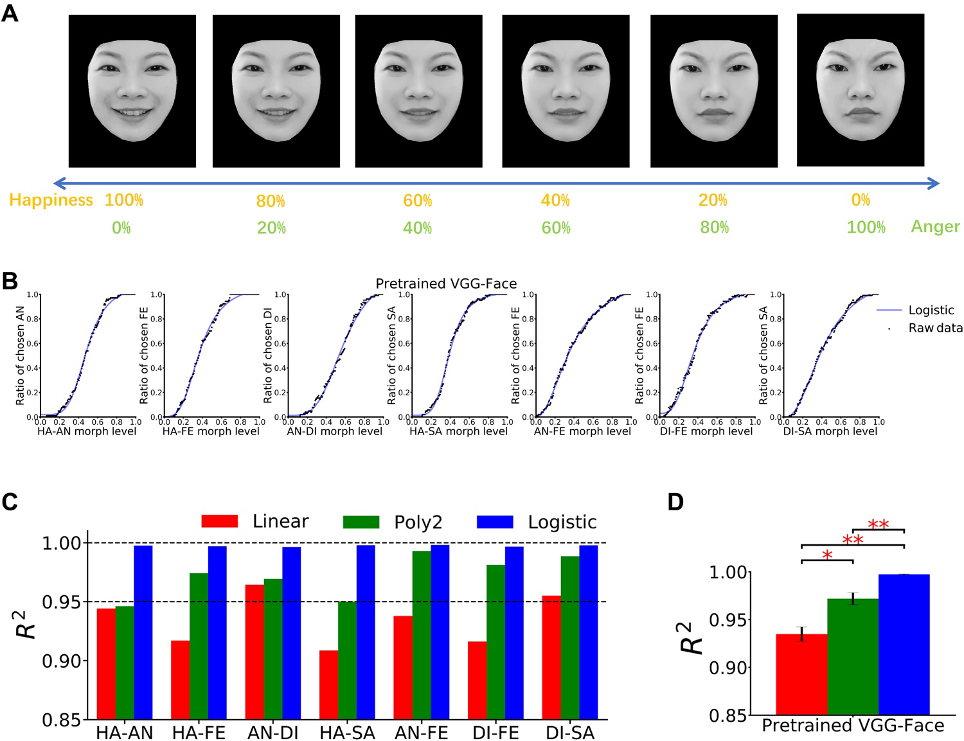
Different from the biological system, DNN allows us to answer the ‘why’ quesiton beyond phenomena description. By altering model architecture, training data, objective function or learning algorithm, we can test the theories that aim to explain behavioral and neural phenomena.
In this study, we also probed expression-selective units in the following networks:
- VGG-16, same architecture with VGG-face, but optimized for object recognition
- to test whether domain-specific or domain-general experience is necessary for facial expression representation
- Randomized VGG-face
- to test the contribution of the architecture
Remarkably, while expression-selective units can be found in both networks, they are not human-like (Fig. 4), which highlights the domain-specific experience for facial expression representation.
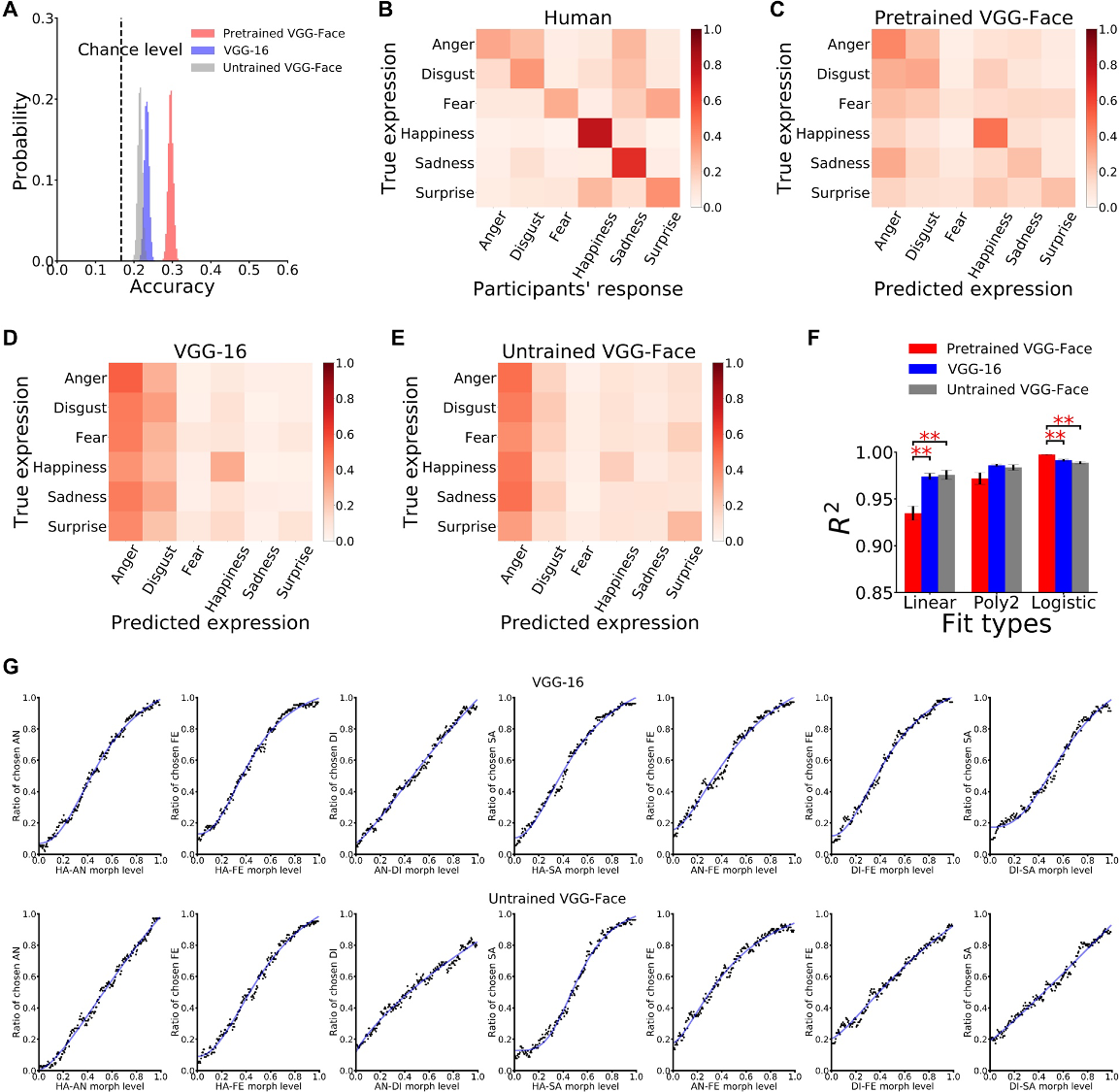
See our work on Science Advances.